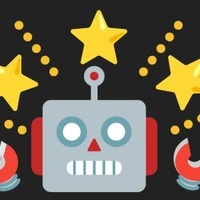
autoevaluator
HF staff
Add evaluation results on the lener_br config and validation split of lener_br
e5afabb
language: | |
- pt | |
tags: | |
- generated_from_trainer | |
datasets: | |
- lener_br | |
metrics: | |
- precision | |
- recall | |
- f1 | |
- accuracy | |
model-index: | |
- name: checkpoints | |
results: | |
- task: | |
name: Token Classification | |
type: token-classification | |
dataset: | |
name: lener_br | |
type: lener_br | |
metrics: | |
- name: F1 | |
type: f1 | |
value: 0.9082022949426265 | |
- name: Precision | |
type: precision | |
value: 0.8975220495590088 | |
- name: Recall | |
type: recall | |
value: 0.9191397849462366 | |
- name: Accuracy | |
type: accuracy | |
value: 0.9808310603867311 | |
- name: Loss | |
type: loss | |
value: 0.1228889599442482 | |
- task: | |
type: token-classification | |
name: Token Classification | |
dataset: | |
name: lener_br | |
type: lener_br | |
config: lener_br | |
split: validation | |
metrics: | |
- name: Accuracy | |
type: accuracy | |
value: 0.9808615356484374 | |
verified: true | |
- name: Precision | |
type: precision | |
value: 0.9853426225094238 | |
verified: true | |
- name: Recall | |
type: recall | |
value: 0.9864880801954342 | |
verified: true | |
- name: F1 | |
type: f1 | |
value: 0.9859150186480775 | |
verified: true | |
- name: loss | |
type: loss | |
value: 0.11563518643379211 | |
verified: true | |
widget: | |
- text: "Ao Instituto M\xE9dico Legal da jurisdi\xE7\xE3o do acidente ou da resid\xEA\ | |
ncia cumpre fornecer, no prazo de 90 dias, laudo \xE0 v\xEDtima (art. 5, \xA7\ | |
\ 5, Lei n. 6.194/74 de 19 de dezembro de 1974), fun\xE7\xE3o t\xE9cnica que\ | |
\ pode ser suprida por prova pericial realizada por ordem do ju\xEDzo da causa,\ | |
\ ou por prova t\xE9cnica realizada no \xE2mbito administrativo que se mostre\ | |
\ coerente com os demais elementos de prova constante dos autos." | |
- text: "Acrescento que n\xE3o h\xE1 de se falar em viola\xE7\xE3o do artigo 114,\ | |
\ \xA7 3\xBA, da Constitui\xE7\xE3o Federal, posto que referido dispositivo revela-se\ | |
\ impertinente, tratando da possibilidade de ajuizamento de diss\xEDdio coletivo\ | |
\ pelo Minist\xE9rio P\xFAblico do Trabalho nos casos de greve em atividade essencial." | |
- text: "Todavia, entendo que extrair da aludida norma o sentido expresso na reda\xE7\ | |
\xE3o acima implica desconstruir o significado do texto constitucional, o que\ | |
\ \xE9 absolutamente vedado ao int\xE9rprete. Nesse sentido, cito Dimitri Dimoulis:\ | |
\ \u2018(...) ao int\xE9rprete n\xE3o \xE9 dado escolher significados que n\xE3\ | |
o estejam abarcados pela moldura da norma. Interpretar n\xE3o pode significar\ | |
\ violentar a norma.\u2019 (Positivismo Jur\xEDdico. S\xE3o Paulo: M\xE9todo,\ | |
\ 2006, p. 220).59. Dessa forma, deve-se tomar o sentido etimol\xF3gico como limite\ | |
\ da atividade interpretativa, a qual n\xE3o pode superado, a ponto de destruir\ | |
\ a pr\xF3pria norma a ser interpretada. Ou, como diz Konrad Hesse, \u2018o texto\ | |
\ da norma \xE9 o limite insuper\xE1vel da atividade interpretativa.\u2019 (Elementos\ | |
\ de Direito Constitucional da Rep\xFAblica Federal da Alemanha, Porto Alegre:\ | |
\ Sergio Antonio Fabris, 2003, p. 71)." | |
## (BERT large) NER model in the legal domain in Portuguese (LeNER-Br) | |
**ner-bert-large-portuguese-cased-lenerbr** is a NER model (token classification) in the legal domain in Portuguese that was finetuned on 20/12/2021 in Google Colab from the model [pierreguillou/bert-large-cased-pt-lenerbr](https://huggingface.co/pierreguillou/bert-large-cased-pt-lenerbr) on the dataset [LeNER_br](https://huggingface.co/datasets/lener_br) by using a NER objective. | |
Due to the small size of the finetuning dataset, the model overfitted before to reach the end of training. Here are the overall final metrics on the validation dataset (*note: see the paragraph "Validation metrics by Named Entity" to get detailed metrics*): | |
- **f1**: 0.9082022949426265 | |
- **precision**: 0.8975220495590088 | |
- **recall**: 0.9191397849462366 | |
- **accuracy**: 0.9808310603867311 | |
- **loss**: 0.1228889599442482 | |
Check as well the [base version of this model](https://huggingface.co/pierreguillou/ner-bert-base-cased-pt-lenerbr) with a f1 of 0.893. | |
**Note**: the model [pierreguillou/bert-large-cased-pt-lenerbr](https://huggingface.co/pierreguillou/bert-large-cased-pt-lenerbr) is a language model that was created through the finetuning of the model [BERTimbau large](https://huggingface.co/neuralmind/bert-large-portuguese-cased) on the dataset [LeNER-Br language modeling](https://huggingface.co/datasets/pierreguillou/lener_br_finetuning_language_model) by using a MASK objective. This first specialization of the language model before finetuning on the NER task allows to get a better NER model. | |
## Blog post | |
[NLP | Modelos e Web App para Reconhecimento de Entidade Nomeada (NER) no domínio jurídico brasileiro](https://medium.com/@pierre_guillou/nlp-modelos-e-web-app-para-reconhecimento-de-entidade-nomeada-ner-no-dom%C3%ADnio-jur%C3%ADdico-b658db55edfb) (29/12/2021) | |
## Widget & App | |
You can test this model into the widget of this page. | |
Use as well the [NER App](https://huggingface.co/spaces/pierreguillou/ner-bert-pt-lenerbr) that allows comparing the 2 BERT models (base and large) fitted in the NER task with the legal LeNER-Br dataset. | |
## Using the model for inference in production | |
```` | |
# install pytorch: check https://pytorch.org/ | |
# !pip install transformers | |
from transformers import AutoModelForTokenClassification, AutoTokenizer | |
import torch | |
# parameters | |
model_name = "pierreguillou/ner-bert-large-cased-pt-lenerbr" | |
model = AutoModelForTokenClassification.from_pretrained(model_name) | |
tokenizer = AutoTokenizer.from_pretrained(model_name) | |
input_text = "Acrescento que não há de se falar em violação do artigo 114, § 3º, da Constituição Federal, posto que referido dispositivo revela-se impertinente, tratando da possibilidade de ajuizamento de dissídio coletivo pelo Ministério Público do Trabalho nos casos de greve em atividade essencial." | |
# tokenization | |
inputs = tokenizer(input_text, max_length=512, truncation=True, return_tensors="pt") | |
tokens = inputs.tokens() | |
# get predictions | |
outputs = model(**inputs).logits | |
predictions = torch.argmax(outputs, dim=2) | |
# print predictions | |
for token, prediction in zip(tokens, predictions[0].numpy()): | |
print((token, model.config.id2label[prediction])) | |
```` | |
You can use pipeline, too. However, it seems to have an issue regarding to the max_length of the input sequence. | |
```` | |
!pip install transformers | |
import transformers | |
from transformers import pipeline | |
model_name = "pierreguillou/ner-bert-large-cased-pt-lenerbr" | |
ner = pipeline( | |
"ner", | |
model=model_name | |
) | |
ner(input_text) | |
```` | |
## Training procedure | |
### Notebook | |
The notebook of finetuning ([HuggingFace_Notebook_token_classification_NER_LeNER_Br.ipynb](https://github.com/piegu/language-models/blob/master/HuggingFace_Notebook_token_classification_NER_LeNER_Br.ipynb)) is in github. | |
### Hyperparameters | |
# batch, learning rate... | |
- per_device_batch_size = 2 | |
- gradient_accumulation_steps = 2 | |
- learning_rate = 2e-5 | |
- num_train_epochs = 10 | |
- weight_decay = 0.01 | |
- optimizer = AdamW | |
- betas = (0.9,0.999) | |
- epsilon = 1e-08 | |
- lr_scheduler_type = linear | |
- seed = 42 | |
# save model & load best model | |
- save_total_limit = 7 | |
- logging_steps = 500 | |
- eval_steps = logging_steps | |
- evaluation_strategy = 'steps' | |
- logging_strategy = 'steps' | |
- save_strategy = 'steps' | |
- save_steps = logging_steps | |
- load_best_model_at_end = True | |
- fp16 = True | |
# get best model through a metric | |
- metric_for_best_model = 'eval_f1' | |
- greater_is_better = True | |
### Training results | |
```` | |
Num examples = 7828 | |
Num Epochs = 20 | |
Instantaneous batch size per device = 2 | |
Total train batch size (w. parallel, distributed & accumulation) = 4 | |
Gradient Accumulation steps = 2 | |
Total optimization steps = 39140 | |
Step Training Loss Validation Loss Precision Recall F1 Accuracy | |
500 0.250000 0.140582 0.760833 0.770323 0.765548 0.963125 | |
1000 0.076200 0.117882 0.829082 0.817849 0.823428 0.966569 | |
1500 0.082400 0.150047 0.679610 0.914624 0.779795 0.957213 | |
2000 0.047500 0.133443 0.817678 0.857419 0.837077 0.969190 | |
2500 0.034200 0.230139 0.895672 0.845591 0.869912 0.964070 | |
3000 0.033800 0.108022 0.859225 0.887312 0.873043 0.973700 | |
3500 0.030100 0.113467 0.855747 0.885376 0.870310 0.975879 | |
4000 0.029900 0.118619 0.850207 0.884946 0.867229 0.974477 | |
4500 0.022500 0.124327 0.841048 0.890968 0.865288 0.975041 | |
5000 0.020200 0.129294 0.801538 0.918925 0.856227 0.968077 | |
5500 0.019700 0.128344 0.814222 0.908602 0.858827 0.969250 | |
6000 0.024600 0.182563 0.908087 0.866882 0.887006 0.968565 | |
6500 0.012600 0.159217 0.829883 0.913763 0.869806 0.969357 | |
7000 0.020600 0.183726 0.854557 0.893333 0.873515 0.966447 | |
7500 0.014400 0.141395 0.777716 0.905161 0.836613 0.966828 | |
8000 0.013400 0.139378 0.873042 0.899140 0.885899 0.975772 | |
8500 0.014700 0.142521 0.864152 0.901505 0.882433 0.976366 | |
9000 0.010900 0.122889 0.897522 0.919140 0.908202 0.980831 | |
9500 0.013500 0.143407 0.816580 0.906667 0.859268 0.973395 | |
10000 0.010400 0.144946 0.835608 0.908387 0.870479 0.974629 | |
10500 0.007800 0.143086 0.847587 0.910108 0.877735 0.975985 | |
11000 0.008200 0.156379 0.873778 0.884301 0.879008 0.976321 | |
11500 0.008200 0.133356 0.901193 0.910108 0.905628 0.980328 | |
12000 0.006900 0.133476 0.892202 0.920215 0.905992 0.980572 | |
12500 0.006900 0.129991 0.890159 0.904516 0.897280 0.978683 | |
```` | |
### Validation metrics by Named Entity | |
```` | |
{'JURISPRUDENCIA': {'f1': 0.8135593220338984, | |
'number': 657, | |
'precision': 0.865979381443299, | |
'recall': 0.7671232876712328}, | |
'LEGISLACAO': {'f1': 0.8888888888888888, | |
'number': 571, | |
'precision': 0.8952042628774423, | |
'recall': 0.882661996497373}, | |
'LOCAL': {'f1': 0.850467289719626, | |
'number': 194, | |
'precision': 0.7777777777777778, | |
'recall': 0.9381443298969072}, | |
'ORGANIZACAO': {'f1': 0.8740635033892258, | |
'number': 1340, | |
'precision': 0.8373205741626795, | |
'recall': 0.914179104477612}, | |
'PESSOA': {'f1': 0.9836677554829678, | |
'number': 1072, | |
'precision': 0.9841269841269841, | |
'recall': 0.9832089552238806}, | |
'TEMPO': {'f1': 0.9669669669669669, | |
'number': 816, | |
'precision': 0.9481743227326266, | |
'recall': 0.9865196078431373}, | |
'overall_accuracy': 0.9808310603867311, | |
'overall_f1': 0.9082022949426265, | |
'overall_precision': 0.8975220495590088, | |
'overall_recall': 0.9191397849462366} | |
```` |