File size: 4,452 Bytes
20418bf 4c6fa67 db9596c 4c6fa67 4d76b14 db9596c 4c6fa67 74e9cd7 4c6fa67 74e9cd7 4c6fa67 ac34889 4c6fa67 28322a9 4c6fa67 28322a9 4c6fa67 ed5aae9 502e506 4c6fa67 caa42e7 4c6fa67 0558c89 4c6fa67 554bde2 caa42e7 554bde2 caa42e7 554bde2 4c6fa67 caa42e7 4c6fa67 b88be3a 4c6fa67 13cd5b9 caa42e7 13cd5b9 caa42e7 4c6fa67 4d76b14 4c6fa67 caa42e7 4c6fa67 caa42e7 4c6fa67 caa42e7 4d76b14 caa42e7 |
1 2 3 4 5 6 7 8 9 10 11 12 13 14 15 16 17 18 19 20 21 22 23 24 25 26 27 28 29 30 31 32 33 34 35 36 37 38 39 40 41 42 43 44 45 46 47 48 49 50 51 52 53 54 55 56 57 58 59 60 61 62 63 64 65 66 67 68 69 70 71 72 73 74 75 76 77 78 79 80 81 82 83 84 85 86 87 88 89 90 91 92 93 94 95 96 97 98 99 100 101 102 103 104 105 106 107 108 109 110 111 112 113 114 115 116 117 118 119 120 121 122 123 124 125 126 127 128 129 130 131 132 |
---
license: apache-2.0
---
# LimaRP-Mistral-7B (Alpaca, flipped instruction experiment)
This is a version of LimaRP for [Mistral-7B-v0.1](https://huggingface.co/mistralai/Mistral-7B-v0.1) with
about 2000 training samples _up to_ 9k tokens length. The second training epoch used a differently arranged
system instruction.
For more details about LimaRP, see the model page for the [previously released v2 version for Llama-2](https://huggingface.co/lemonilia/limarp-llama2-v2).
Most details written there apply for this version as well. Generally speaking, LimaRP is a longform-oriented, novel-style
roleplaying chat model intended to replicate the experience of 1-on-1 roleplay on Internet forums. Short-form,
IRC/Discord-style RP (aka "Markdown format") is not supported yet. The model does not include instruction tuning,
only manually picked and slightly edited RP conversations with persona and scenario data.
## Prompt format
Same as before. It uses the [extended Alpaca format](https://github.com/tatsu-lab/stanford_alpaca),
with `### Input:` immediately preceding user inputs and `### Response:` immediately preceding
model outputs. While Alpaca wasn't originally intended for multi-turn responses, in practice this
is not a problem; the format follows a pattern already used by other models.
```
### Instruction:
Character's Persona: {bot character description}
User's Persona: {user character description}
Scenario: {what happens in the story}
Play the role of Character. You must engage in a roleplaying chat with User below this line. Do not write dialogues and narration for User.
### Input:
User: {utterance}
### Response:
Character: {utterance}
### Input
User: {utterance}
### Response:
Character: {utterance}
(etc.)
```
You should:
- Replace all text in curly braces (curly braces included) with your own text.
- Replace `User` and `Character` with appropriate names.
### Message length control
Inspired by the previously named "Roleplay" preset in SillyTavern, with this
version of LimaRP it is possible to append a length modifier to the response instruction
sequence, like this:
```
### Input
User: {utterance}
### Response: (length = medium)
Character: {utterance}
```
This has an immediately noticeable effect on bot responses. The lengths using during training are:
`micro`, `tiny`, `short`, `medium`, `long`, `massive`, `huge`, `enormous`, `humongous`, `unlimited`.
**The recommended starting length is medium**. Keep in mind that the AI can ramble or impersonate
the user with very long messages.
The length control effect is reproducible, but the messages will not necessarily follow
lengths very precisely, rather follow certain ranges on average, as seen in this table
with data from tests made with one reply at the beginning of the conversation:

Response length control appears to work well also deep into the conversation. **By omitting
the modifier, the model will choose the most appropriate response length** (although it might
not necessarily be what the user desires).
## Suggested settings
You can follow these instruction format settings in SillyTavern. Replace `medium` with
your desired response length:
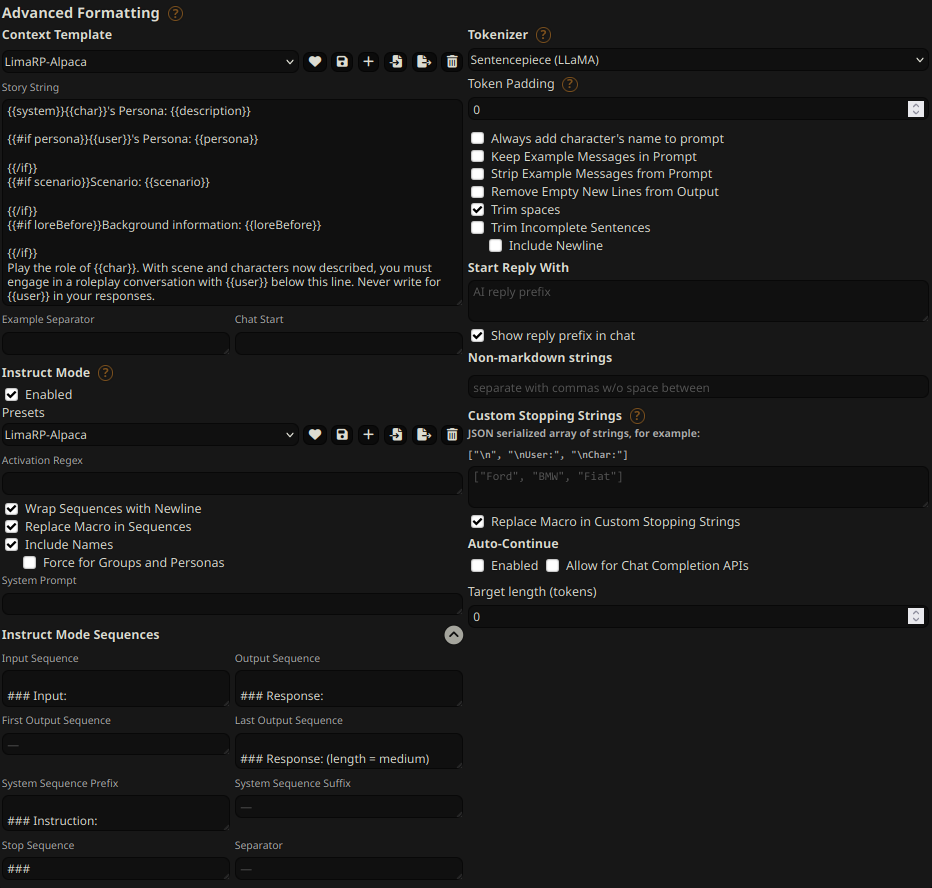
## Text generation settings
These settings could be a good general starting point:
- TFS = 0.92
- Temperature = 0.70
- Repetition penalty = ~1.1
- Repetition penalty range = ~2048
- top-k = 0 (disabled)
- top-p = 1 (disabled)
## Training procedure
[Axolotl](https://github.com/OpenAccess-AI-Collective/axolotl) was used for training
on 4x NVidia A40 GPUs.
The A40 GPUs have been graciously provided by [Arc Compute](https://www.arccompute.io/).
### Training hyperparameters
Although 1 training epoch was used, the underlying data comprised data repeated twice
in slightly different formats.
- learning_rate: 0.0003
- lr_scheduler: constant_with_warmup
- noisy_embedding_alpha: 5
- num_epochs: 1
- sequence_len: 8750
- lora_r: 256
- lora_alpha: 16
- lora_dropout: 0.05
- lora_target_linear: True
- bf16: True
- fp16: false
- tf32: True
- load_in_8bit: True
- adapter: lora
- micro_batch_size: 1
- gradient_accumulation_steps: 1
- warmup_steps: 10
- optimizer: adamw_torch
- flash_attention: true
- sample_packing: true
- pad_to_sequence_len: true
Using 4 GPUs, the effective global batch size would have been 4.
### Training loss graph
 |